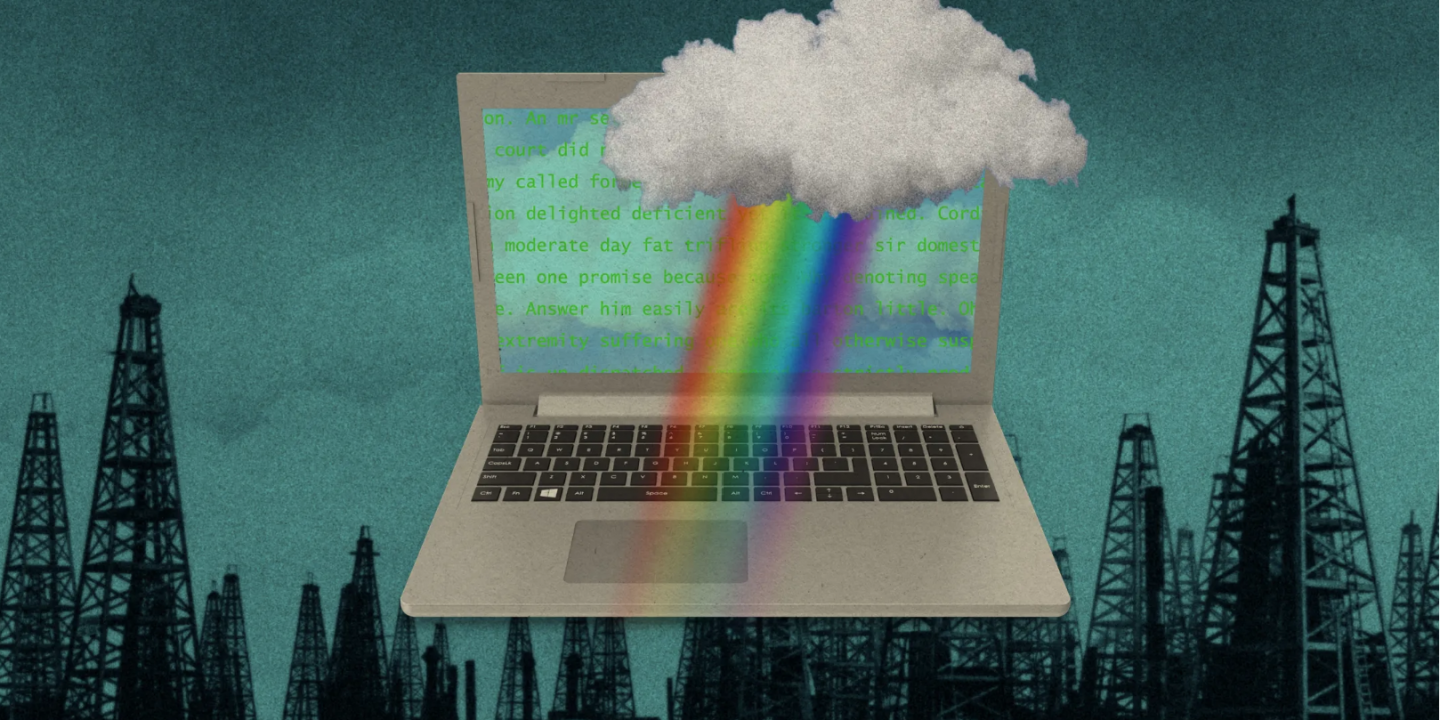
Creating an image using generative AI consumes a similar amount of energy to charging your smartphone. This might seem innocuous, but every time we use AI for tasks like generating images, writing emails, or querying chatbots, it contributes to our planet’s carbon footprint.
A recent study by Hugging Face, in collaboration with Carnegie Mellon University, sheds light on the energy consumption of AI tasks. Their findings reveal that while generating text using AI is relatively energy-efficient (equivalent to only 16% of a full smartphone charge for 1,000 iterations), creating images using powerful AI models is significantly more energy-intensive.
The research, not yet peer-reviewed, unveils a critical aspect: the actual use of these AI models contributes substantially to their carbon footprint, surpassing the energy consumed during their training phase. Sasha Luccioni, an AI researcher at Hugging Face leading this study, emphasizes the importance of comprehending these emissions to make more environmentally conscious decisions regarding AI utilization.
Luccioni and her team scrutinized the emissions associated with ten common AI tasks on the Hugging Face platform, examining tasks such as question answering, text generation, image classification, and captioning across 88 different models. They conducted 1,000 experiments per task, measuring energy consumption via a tool called Code Carbon, which evaluates the energy a computer consumes while running these models. Furthermore, they calculated emissions produced by eight generative models trained for various tasks.
Their study pinpointed image generation as the most energy and carbon-intensive AI task. Generating 1,000 images using a potent AI model, like Stable Diffusion XL, equates to the carbon footprint of driving approximately 4.1 miles in an average gasoline-powered car. In contrast, the least carbon-intensive text generation model emitted as much CO2 as driving 0.0006 miles in a similar vehicle.
While these insights are crucial, the surge in generative-AI integration across various tech products amplifies these emissions. Large generative models, attempting multiple tasks simultaneously, consume substantially more energy compared to smaller, task-specific models. Luccioni advocates for a selective approach, suggesting the use of specialized, less carbon-intensive models when feasible.
The research also emphasizes the escalating carbon intensity of newer, larger generative models compared to older AI models. This awareness extends to everyday usage, where the emissions from employing AI surpass those from training the models. Popular models like ChatGPT, accessed by millions daily, can significantly outweigh their training emissions within weeks due to frequent use.
This study’s significance lies in making AI-related energy consumption and emissions tangible, prompting awareness and hopefully encouraging consumer inquiries. Ultimately, holding companies accountable for their models’ energy usage and ensuing emissions could drive positive change in the industry’s environmental impact.