Unlock Business Opportunities With Data Analytics
Our Machine Learning Engagement Models
Choose from our different engagement models that suit your business needs.
Strategy
Architecture
Research & Development
Consulting
Integration
Support & Maintenance
Our Machine Learning Development Capabilities
We are dedicated to delivering high-quality technology solutions backed by best practices and design patterns
Predictive Analysis
Deep Learning
Video Analytics
Image Analytics
Audio Analytics
Natural Language Processing
Business Intelligence
Data Analytics
Why Machine Learning Service At Vegavid?
With Machine learning service at Vegavid now it's possible for design systems to be capable of exhibiting uncanny human-like thinking.
Machine Learning Use Cases We Cover
Here Is How We Achieve Goals At Vegavid
Data Acquisition
We begin with defining business needs and analyzing the best optimization of machine learning solutions.
Data Preparation
We clean the data to enhance its quality and is easy to process or analyze.
Data Modelling
We build and train machine learning algorithms to predict the features' labels, tuning them for the business need.
Model Deployment
Once you’re happy with the evaluation, we go ahead with ML model deployment .
Tools And Technologies We Use

Tensorflow

Keras
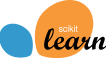
Scikit learn

Open CV
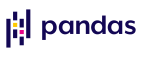
Pandas

NLTK
FAQs
Do you also have these questions?
Machine learning is a branch of artificial intelligence (AI) and computer science which focuses on the use of data and algorithms to imitate the way that humans learn, gradually improving its accuracy.
Machine learning is one of the most trending technologies, and it's already present in many business applications. However, if you are new to machine learning in business, you have come to the right place. Vegavid Technology is the best machine learning development company to help companies analyze their needs and suggest the best possible machine learning solutions.
Machine learning facilities business for solving the following problems:
- Make predictions based on historical data.
- Create models that can be used to guide decision-making.
There are many ways to get started with machine learning development for business. One of the easiest ways is to use existing machine learning frameworks. The most popular frameworks are Scikit-learn and Tensorflow, which are open source and free to use. Talk to Vegavid machine learning experts to know how your business can benefit from machine learning services.
Machine learning infrastructure includes the resources, processes, and tooling needed to develop, train, and operate machine learning models. It is sometimes referred to as AI infrastructure or a component of MLOps. ML infrastructure supports every stage of machine learning workflows. It enables data scientists, engineers, and DevOps teams to manage and operate the various resources and processes required to train and deploy neural network models.
When it comes to finding the best infrastructure for machine learning development, there are numerous factors you must evaluate. On the one hand, your infrastructure should offer sufficient storage space and high network performance. On the other hand, choosing a scalable machine learning platform that supports multiple data types is also crucial. It is also essential to pick a provider with an easy-to-use platform accessible anytime and anywhere. The infrastructure you choose should provide you with everything you need to succeed.
This depends on the complexity of the application. However, most ML projects are broken down into task-related components. For example, a typical ML project may involve data cleansing, data preparation, feature extraction, model building, evaluation, and deployment. Each of these components requires a certain amount of time, depending on the project. For example, data cleaning can take a few hours to a few days. However, it is a very straightforward process and does not require much time. On the other hand, the model building can be a more complex process, which can take multiple weeks. Again, this depends on the type of model you build, the number of features, and the dataset size.
Machine learning is a complex topic, and developing machine learning applications can be divided into several steps. If you want to build a machine learning application, there are three steps that you need to follow.
- Collect Data: In this process data is collected from various sources such as APIs.
- Prepare the Data: At this stage data can be pre processed by normalising , eliminating duplicates and error corrections.
- Choose Model: There are several models that you can choose based on your requirement. You will use algorithms of prediction ,linear regression and clustering.
- Evaluation: During Evaluation you will have to check the machine created against your evaluation data set containing inputs that the model does not know and verify the precision of the already trained model.
- Parameter Tuning: If the evolution did not deliver good predictions and the precision is not minimal desired . It is possible that you have overfitting or underfitting problems and you must return to the training step without making a new configuration of parameters in your model.
- Prediction of Inference: You can now use your Machine Learning model inferring results in real life scenarios.