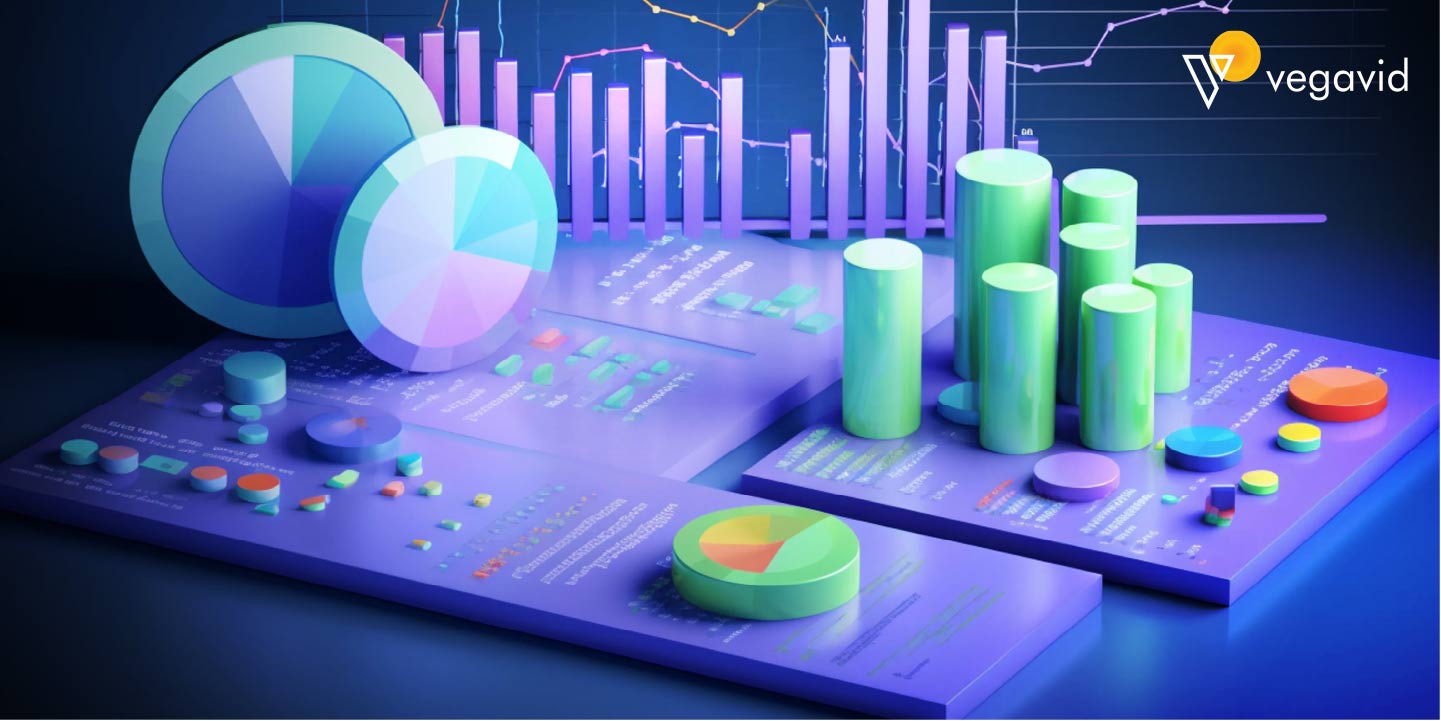
Exploratory Data Analysis (EDA) is a crucial phase in data analysis that unveils valuable insights. Within this process, new revelations may emerge, such as hidden patterns, outliers, or unexpected correlations. EDA can provide a deeper understanding of the data, shedding light on variables that significantly impact the outcome. It can identify data quality issues, helping researchers refine their datasets. Moreover, EDA can suggest potential directions for further investigation, formulating hypotheses, or guiding the selection of appropriate statistical methods. In essence, EDA serves as the initial step in data exploration, often revealing unanticipated aspects that can significantly influence subsequent analysis and decision-making. Data can offer incredible insights if we take the time for exploratory data analysis. Let’s dive in and see what hidden gems we might uncover!
A Positive Path Of Discovery
Rather than jumping to conclusions, an exploratory approach allows us to discover relationships and patterns in a spirit of curiosity rather than judgment. Each new finding adds to our understanding without prejudging what’s essential. By maintaining an open mind, unexpected revelations may surface. A positive path of discovery embodies the journey of exploration and learning with optimism and open-mindedness. It’s a mindset that seeks to uncover knowledge, growth, and opportunities in every experience. This path encourages curiosity, resilience, and a willingness to embrace challenges as valuable learning opportunities. It promotes the understanding that setbacks and failures are stepping stones toward personal and professional development. On a positive path of discovery, individuals adapt to change, foster creativity, and build meaningful connections. It’s a transformative journey that fuels personal and collective progress, promising a brighter future through continuous exploration, innovation, and the unwavering pursuit of knowledge.
Initial Data Dive
Recently a project was completed that involved collecting lots of participant survey responses. Now it’s time to shift gears from gathering information to exploring what insights might emerge from a closer look—no hypotheses—just a willingness to learn.
First, start with basic descriptive stats to understand distributions and variability for each variable. Things like ranges, means, and measures of central tendency can highlight any potential outliers or skewing that warrant investigation. From there, visualizing relationships through simple graphs is a fun way to spot interesting correlations across different demographic groups. The initial data dive was an exhilarating journey into the depths of information, a plunge into the vast sea of raw data. It was a process of exploration to immerse yourself in the numbers, texts, and figures that held the potential to reveal hidden insights. Starting with an array of questions and hypotheses, it is combed through the dataset, looking for patterns, anomalies, and meaningful correlations. With each discovery, it is gained a deeper understanding of the subject matter. The initial data dive was a foundational step when data transformed into knowledge, laying the groundwork for more in-depth analysis and generating valuable
Preliminary Patterns Appearing
Some early trends take shape as we analyze response frequencies and distributions. For example, participant enjoyment appears moderately high across most activities but spikes way up for one. Regional differences also show up prominently for specific opinions. Nothing is conclusive yet, just intriguing breadcrumbs worth following to see where they lead. The goal is to let the data guide discovery rather than forcing preconceived notions onto it. As it is delved deeper into the data, preliminary patterns begin to emerge, like faint brushstrokes on a canvas slowly revealing a picture. It was a moment of intrigue and curiosity as specific trends, associations, and regularities became apparent. These early glimpses offered a glimpse into the underlying structure of the dataset, hinting at potential relationships and critical variables. While inconclusive, these preliminary patterns were a foundation for further exploration and analysis. They catalyzed more refined questions and a sense of excitement as they recognized the data’s potential to unveil meaningful insights and contribute to a broader understanding of the subject at hand.
Deeper Dives Reveal More Connections
As analyses expand to include additional variables, new correlations are emerging. For instance, comparing enjoyment ratings to participation frequency revealed those who engage more regularly in a given activity tend to like it significantly more than their less frequent counterparts. Meanwhile, cross-tabbing demographic traits against responses exposed some thought-provoking disparities between certain groups worth unpacking further. The possibilities unfolding through exploratory data analysis are getting more exciting!
As you embarked on deeper dives into the data, a rich tapestry of connections and relationships unfolded. With each layer of analysis, you have uncovered more intricate patterns previously concealed beneath the surface. It was like solving a complex puzzle, where each piece provided greater clarity and context. The data revealed not only correlations but also potential causations and outliers of significance. This iterative exploration process added depth and nuance to my understanding, empowering me to draw more robust conclusions and make informed decisions. These revelations demonstrated the invaluable nature of thorough data analysis, where each deeper dive unveiled a more comprehensive picture of the subject matter.
Sharing Preliminary Findings
While conclusions must wait until diving deeper, it is eager to chat with colleagues about early patterns identified. Getting their perspectives may spark additional questions or a layer of contextual knowledge is needed to gain. Together, we can brainstorm ways to probe emerging connections in subsequent analyses. Collaboration makes data exploration even more enjoyable and increases the chances of stumbling upon novel insights. With their expertise lending new angles, who knows what hidden gems might surface?
Sharing preliminary findings is a crucial step in the data analysis process. It involves conveying initial insights, trends, and discoveries to relevant stakeholders, team members, or collaborators. This open communication promotes transparency and enables others to offer input and perspective. It can serve as a checkpoint for the validity of findings and provide an opportunity for collective interpretation. Sharing preliminary results is an essential practice in research and decision-making contexts, allowing for adjustments, clarifications, and the alignment of objectives. It fosters collaboration and ensures that the analysis stays on track, leading to more robust and reliable conclusions as the project progresses.
Benefits Of Exploratory Data Analysis
Exploratory Data Analysis (EDA) is a fundamental component of data science and analytics that offers many benefits. In this process, data analysts explore and dissect datasets to unveil hidden patterns, relationships, and potential outliers. EDA is a vital preliminary step before more advanced statistical analyses or model building, and its benefits are manifold.
First and foremost, EDA helps researchers gain a deep understanding of the dataset. By visualizing the data, summarizing statistics, and identifying data distributions, analysts can grasp the essential characteristics of the information at hand. This understanding is crucial because it informs the subsequent analysis and helps researchers tailor their approaches to the specific nature of the data.
EDA often leads to discovering patterns and relationships that might not be immediately apparent. These insights can provide a unique perspective on the subject matter and guide further exploration. For example, in a dataset related to customer purchasing behavior, EDA might reveal that certain products are frequently bought together, pointing to potential cross-selling opportunities for a business.
Moreover, EDA can identify outliers or anomalies in the data. These unusual data points can indicate errors, fraud, or other essential phenomena. By identifying and understanding outliers, analysts can take appropriate actions, such as data cleaning or further investigation. For instance, in financial data, unusual transaction amounts might be flagged for fraud detection.
Exploratory Data Analysis aids in the formulation of hypotheses and research questions. As patterns and trends emerge, researchers can generate new questions and refine their beliefs. For instance, in a healthcare dataset, EDA might reveal a strong association between certain lifestyle factors and the incidence of a particular disease, prompting further investigation into causation.
EDA is an excellent tool for data preprocessing. By understanding the data’s distribution and characteristics, analysts can make informed decisions about data transformations, scaling, and handling missing values. For example, EDA might show that a variable follows a non-normal distribution, suggesting that a logarithmic transformation would be appropriate.
Another significant benefit of EDA is its ability to provide actionable insights. For businesses, this can translate into more effective decision-making. For example, a retailer might use EDA to analyze customer purchase data and discover that a particular demographic tends to shop during specific times. This information can lead to targeted marketing strategies and improved customer experiences.
EDA is not limited to structured data; it can also be applied to unstructured data, such as text or images. By employing techniques like text mining or image recognition, analysts can uncover valuable information and sentiment analysis. In the context of social media data, EDA might reveal sentiment trends around a particular product or topic, helping businesses gauge public opinion.
Furthermore, EDA is an indispensable tool for data visualization. It allows analysts to create charts, graphs, and interactive dashboards that make complex data more accessible and understandable. Visualizations can be used for internal decision-making or to communicate findings to stakeholders. It is valuable in data-driven storytelling, where visual representations enhance the impact of data-driven narratives.
It can be said that Exploratory Data Analysis is a critical phase in data analysis, offering many benefits. From gaining a deep understanding of the dataset to uncovering hidden patterns, identifying outliers, and formulating hypotheses, EDA is a versatile tool that guides researchers and analysts in making informed decisions. Whether applied to structured or unstructured data, its actionable insights and visualization capabilities empower data-driven decision-making, making it an essential step toward valuable discoveries.
Conclusion
Exploratory Data Analysis (EDA) is an indispensable cornerstone of the data analysis process, offering valuable insights and benefits. EDA provides a profound understanding of data through visualization and summary statistics, serving as the compass for further analysis. It unveils hidden patterns, relationships, and outliers, guiding researchers to formulate hypotheses and refine their research questions. The actionable insights derived from EDA have a significant impact on decision-making, both in business and research contexts. These insights empower organizations to create targeted strategies, streamline operations, and enhance customer experiences. Moreover, EDA is adaptable, making it applicable not only to structured data but also to unstructured data, such as text and images, further broadening its utility.
As data analysis continues to play an increasingly pivotal role in decision-making across various domains, the significance of EDA cannot be overstated. It not only streamlines the data analysis process but also facilitates effective communication of insights through data visualization. In essence, EDA is a powerful tool that guides the way toward discoveries, informed decisions, and a deeper understanding of the complex data landscapes of today’s world.