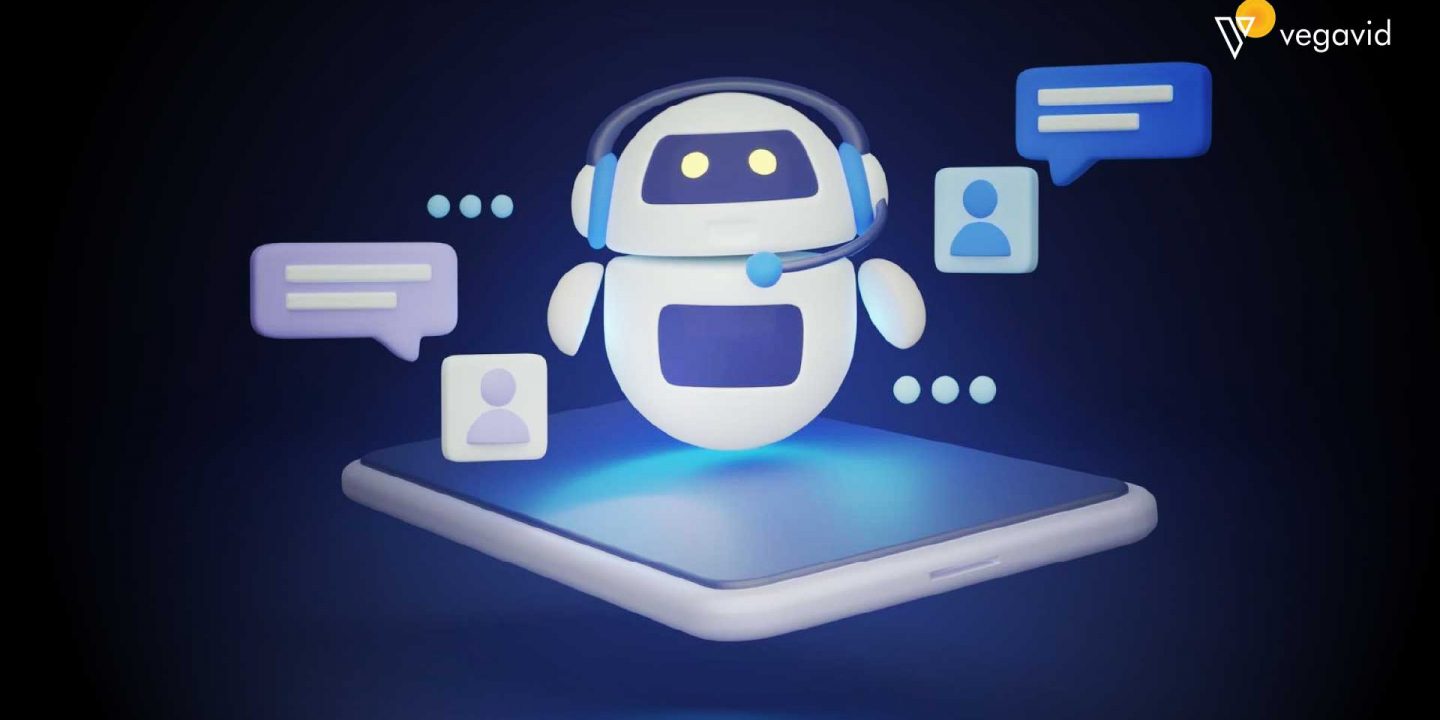
Conversational AI has advanced tremendously in recent years. Systems like ChatGPT can understand natural language and respond coherently. However, most current chatbots have limited capabilities when it comes to visual elements. Visual ChatGPT promises to take conversational AI to new heights by incorporating computer vision. It can understand images, videos, and other visual content in addition to text. In this blog post, we will explore the features of Visual ChatGPT, how it works behind the scenes, and its potential applications across industries. Visual ChatGPT marks an exciting new phase for human-AI interaction with its multi-modal approach.
What is Visual ChatGPT?
Visual ChatGPT is an AI model that builds upon ChatGPT’s conversational abilities with the addition of computer vision skills. Unlike text-only dialogue systems, Visual ChatGPT can understand visual content like images, videos, and maps during a conversation. It uses a combination of natural language processing and computer vision technologies to undergo multi-modal learning from massive language-image datasets. This helps the model develop joint representations for language and vision to truly comprehend the context of any discussion involving visual media. With visual ChatGPT, humans can have natural conversations with an AI assistant and also perform tasks like asking questions about objects in photos, getting relevant information from maps, and discussing and drawing inferences from visual content. This expands ChatGPT’s functionalities to a new multi-modal level.
Features of Visual ChatGPT
Here are the features of visual ChatGPT:
Multi-modal input
One of the key features of Visual ChatGPT is its ability to understand multi-modal input. Unlike traditional text-based chatbots, Visual ChatGPT can comprehend both images and text simultaneously during a conversation. This allows it to interact with users in a much more natural way similar to human conversations. Users can describe an image they want to share or ask the bot a question about an image by uploading it into the chat.
The model then processes both the user’s textual message alongside the visual features extracted from the image using state-of-the-art computer vision models. By fusing this multi-modal data, Visual ChatGPT can derive deeper contextual understanding compared to only analyzing text or images individually. It can then generate response messages that are aware of and accurately aligned with both the verbal and visual information provided by the user.
Image embedding
To process images during conversations, Visual ChatGPT relies on advanced image embedding techniques. It uses pre-trained computer vision models to encode any images into dense numeric vectors, also known as embeddings. These embeddings compactly represent the various visual concepts and features detected in the images by the vision models. Concepts like objects, scene types, and colors are captured in the embeddings.
Through deep learning, the vision models have learned to map vastly different images to similar embedded representations if they contain similar visual contents. Visual ChatGPT can then understand the images by analyzing these embeddings instead of the raw pixels. The embeddings allow it to incorporate the visual context into its text understanding and generation capabilities.
Object recognition
The key capability of Visual ChatGPT is object recognition within images. Using large pre-trained computer vision models, it can visualize and understand the various objects present in the images users provide. During encoding, the vision models generate embedded representations that encode details about the recognized objects like cars, people, furniture, etc. Their types, positions, and other properties are captured in the embeddings. This allows Visual ChatGPT to comprehend the scene or situation depicted through object recognition. It can then incorporate this understanding of the objects into how it processes and responds to the user’s textual inputs. Object recognition greatly enhances its ability to hold visually-aware conversations.
Contextual understanding
The most impressive aspect of Visual ChatGPT is its ability to develop contextual understanding from both text and images. By processing the multimodal inputs simultaneously through attention mechanisms and fused representations, it can understand how the language used and the visual content depicted interrelate. This allows it to derive deeper meaning from the combined inputs rather than analyzing them separately.
For example, it can understand if the objects mentioned in the text match those seen in images. This unified processing of visual and linguistic context enables Visual ChatGPT to hold more natural conversations that effectively integrate verbal and non-verbal information. It can then generate responses that accurately address the overall context established through both channels.
Large-scale training
A key factor that allows Visual ChatGPT to achieve its powerful multimodal abilities is its massive scale of training. It was trained on huge datasets containing billions of examples of text, images, and their alignments. This exposure to broad and diverse data helped the model establish robust associations between various visual and textual concepts.
During training, it learned intricate relationships between objects, scenes, and language by analyzing countless paired examples. Pretraining on such large-scale data endowed Visual ChatGPT with strong foundational skills for understanding context across modalities. This comprehensive pretraining equips it to engage in knowledgeable conversations that seamlessly bridge between the visual and textual domains.
Role of Visual Foundation Models in Visual ChatGPT
Visual foundation models like CLIP play an important role in enabling Visual ChatGPT’s multimodal capabilities. These models are trained on massive image-text datasets to learn visual representations and understanding. They use a similar objective as language models like BERT to learn contextual relationships between images and text. In Visual ChatGPT, CLIP acts as the visual encoder – it embeds input images into a high-dimensional visual vector space.
This allows mapping images into the same semantic space that ChatGPT operates in for text. The visual encodings from CLIP feed into Visual ChatGPT along with the textual input. This allows the model to fuse both modalities and develop a unified contextual understanding. With representations from powerful visual foundations, Visual ChatGPT can understand images almost as well as natural language.
How does Visual ChatGPT work?
Here are the key points about how Visual ChatGPT works:
- Input Processing: When a user sends a message containing both text and an image, Visual ChatGPT first processes these two modes of input separately.
- Textual Encoding: It encodes the textual content using a Transformer encoder to generate contextualized representations of the words and sentences.
- Image Encoding: A pre-trained computer vision model embeds the image into a dense vector representation encoding visual concepts and objects.
- Multimodal Fusion: The text and image embeddings are fused through attention mechanisms to create a unified multimodal representation.
- Decoding: A Transformer decoder integrates information from the multimodal encoding to generate a natural response that addresses both the textual query and visual context.
- Output Generation: Visual ChatGPT produces a text response demonstrating its understanding of the relationship between the image and text. It can also generate additional relevant images as part of its response.
Use cases of Visual ChatGPT
Here are some potential use cases for Visual ChatGPT:
Customer service
Visual ChatGPT has immense potential to improve customer service interactions and help organizations provide faster resolution to customer issues. With its ability to understand images, Visual ChatGPT can analyze photos or screenshots shared by customers to better understand the nature of technical problems. For example, if a customer messages a company’s support account on social media with an image of an error message on their device, Visual ChatGPT can accurately recognize the error code and provide the agent with relevant troubleshooting steps. This allows the agent to quickly resolve the issue without prolonged back-and-forth communication. Visual ChatGPT’s image recognition and contextual response capabilities based on visual and textual inputs can help enhance customer satisfaction by reducing resolution times.
E-commerce
Visual ChatGPT has the potential to profoundly improve online shopping experiences in the e-commerce industry. It could provide personal shopper assistance to customers directly through websites and apps. Customers would be able to chat with Visual ChatGPT and send photos of products they need help finding. The AI would identify the items in the images, and provide product details and recommendations of similar items. This makes the shopping process more intuitive for visual shoppers. Customers may also snap photos of what they currently own and get suggestions on items that match or coordinate well. By understanding images in addition to text, Visual ChatGPT aims to make online shopping as seamless as interacting with a real salesperson.
Social media
On social media platforms, a large amount of content is visual such as photos and videos. Visual ChatGPT can enhance user experience on these sites by analyzing image-based posts and providing useful context. For photos uploaded by users, Visual ChatGPT can automatically caption posts by identifying objects, scenes, or people depicted. It can also fetch related information from its knowledge base to enrich photo descriptions. This helps users understand image posts better. Visual ChatGPT’s ability to understand visuals also allows it to answer questions from users about posts more comprehensively. With its multi-modal approach, conversations on social networks could become more engaging and informative.
Healthcare
Visual ChatGPT has applications in the healthcare industry to improve diagnosis and patient understanding. Doctors could leverage its computer vision capabilities by uploading medical scans and images during virtual consultations. The AI has the potential to analyze images, make preliminary observations about potential abnormalities, and suggest differentials to consider. This would help physicians arrive at diagnoses faster. Patients may also use Visual ChatGPT to better understand their conditions by engaging in visual conversations. They could upload images of symptoms or pages from educational materials, and the chatbot would provide contextual responses and explanations based on its analysis. Overall, it may help enhance clinical decisions and patient education.
Education
In the field of education, Visual ChatGPT can serve as a powerful supplement to traditional teaching methods. It could help students learn complex topics that involve visual components more effectively. Students may converse with Visual ChatGPT by sending photos of diagrams, charts, or experiments for clarification on concepts. The AI is capable of identifying different elements in images and providing detailed descriptions to explain processes. Teachers could also utilize it as a visual teaching assistant that engages with learning materials like illustrations, notes, or lab samples shared by students. Its multimodal question answering would help address conceptual queries involving both text and visual information. Overall, Visual ChatGPT aims to make learning more intuitive and visual.
Conclusion
Visual ChatGPT demonstrates how conversational AI can be enhanced by leveraging state-of-the-art computer vision models. Its ability to comprehend visual inputs along with text allows for richer contextual responses. Various sectors like customer service, e-commerce, education, and healthcare stand to benefit from such a visual chatbot. While the full capabilities of Visual ChatGPT are yet to be seen, it presents an optimistic vision of how human-AI dialogue might evolve to feel more natural. By understanding both language and images, Visual ChatGPT could completely transform how people and machines converse in the future. The next generation of conversational AI is here.