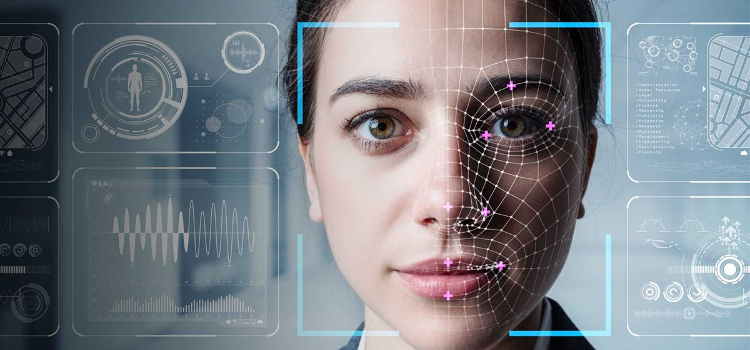
Artificial Intelligence (AI) is a broad field encompassing various technologies and approaches to simulate human intelligence. Generative AI is a subset of AI that specifically focuses on creating content or data. Let’s explore the differences between generative AI and AI as a whole.
AI (Artificial Intelligence)
Definition: AI refers to the development of computer systems or algorithms that can perform tasks typically requiring human intelligence. These tasks include problem-solving, decision-making, language understanding, perception, and learning.
Characteristics:
- Machine Learning: AI often involves machine learning techniques, where algorithms learn from data to improve their performance over time. This includes supervised learning, unsupervised learning, and reinforcement learning.
- Task-Specific: AI can be task-specific or narrow AI, designed to excel in a particular area. For example, AI chatbots, image recognition systems, and recommendation engines are specific applications of AI.
- Rules and Logic: AI systems can also be rule-based, where they follow predefined sets of rules and logic to make decisions or provide answers. This approach is common in expert systems.
- Data-Driven: Many AI applications rely on data to make predictions or decisions. The quality and quantity of data play a crucial role in the effectiveness of AI models.
- Real-World Applications: AI is used in various industries, including healthcare, finance, transportation, and entertainment, to automate tasks, improve efficiency, and enhance user experiences.
Generative AI
Definition: It is also known as generative models, refers to AI systems that can generate new data, content, or information. These models learn patterns and structures from existing data and use that knowledge to produce novel outputs.
Characteristics:
- Content Creation: It is primarily focused on content creation, such as text, images, audio, and even video. It can generate realistic content that mimics human creations.
- Examples: Prominent examples of generative AI models include GPT-3 (Generative Pre-trained Transformer 3) for natural language generation and deep learning-based models like GANs (Generative Adversarial Networks) for image generation.
- Data Synthesis: Generative AI can synthesize data that appears as if it were created by humans. This has applications in creative fields, content generation, and even generating realistic but fictitious data for research purposes.
- Innovation: Generative AI can foster innovation by automating creative tasks and aiding artists, writers, and designers in their work.
- Challenges: Ethical considerations and concerns about the potential misuse of generative AI, such as deepfakes and misinformation, are ongoing challenges in the field.
Conclusion
In summary, AI is a broad field encompassing various technologies and applications designed to replicate human intelligence across different domains. Generative AI, on the other hand, is a specialized subset of AI that focuses on creating content or data, often by learning patterns and structures from existing information. Both AI and generative AI have diverse real-world applications and hold the potential to transform industries and the way we interact with technology.