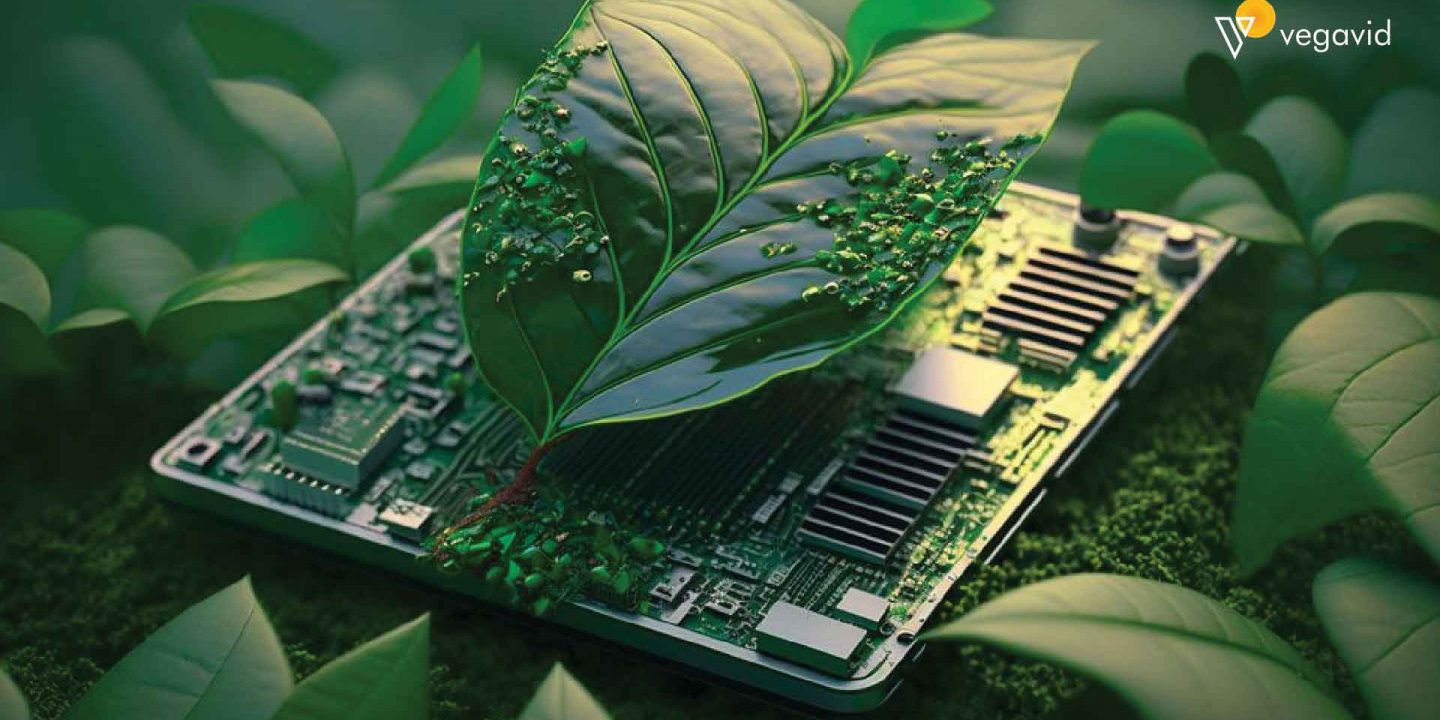
Artificial Intelligence (AI) is everywhere—powering your favorite apps, smart devices, cars, and even the weather forecasts you check daily. But behind the scenes, AI has a dirty secret: it can be energy-hungry and carbon-heavy.
That’s where Green AI comes in.
Green AI refers to energy-efficient AI development that reduces power consumption, cuts down emissions, and supports sustainable technology growth. As climate change accelerates, Green AI is quickly becoming a powerful solution for building eco-conscious digital systems.
Why Does Green AI Matter?
You might think AI is just code. But in reality, training an AI model like ChatGPT or an image generator can consume as much energy as five cars driving for a year. Shocking, right?
According to a 2024 forecast, AI systems could use over 85 TWh (terawatt-hours) annually by 2026—more than the entire power consumption of some countries.
This rising energy demand has made Green AI not just a trend but a necessity.
Key Features of Green AI
Let’s break down what makes AI “green”:
1. Energy-Efficient Algorithms
Green AI models are built to use fewer resources, run faster, and maintain high accuracy. Techniques like model pruning, quantization, and distillation help reduce the carbon footprint of training deep learning systems.
2. Eco-Friendly AI Hardware
Traditional GPUs and servers eat up energy. Green AI uses energy-saving processors, low-voltage chips, and neuromorphic computing—hardware that mimics the human brain’s efficiency.
Example: IBM’s TrueNorth chip runs powerful AI while using 1,000x less power than a typical graphics card.
3. Sustainable Data Centers
The backbone of AI is the data center. But many data centers rely on fossil fuels. Green AI promotes renewable-powered AI operations, like Google’s carbon-neutral data centers or Microsoft’s AI on clean energy.
Benefits of Green AI
Green AI isn’t just good for the planet — it’s also good for your bottom line, your users, and your reputation. Here are benefit of Green AI:
Benefit | Description | Related Keywords |
---|---|---|
Reduced Energy Consumption | Green AI models consume significantly less electricity compared to traditional AI systems. | Energy-efficient AI, low-power machine learning, sustainable models |
Lower Operational Costs | Optimized algorithms and efficient hardware reduce cloud compute costs and infrastructure overhead. | Cost-effective AI, efficient algorithm deployment |
Supports Sustainable Tech | Aligns with global green tech initiatives and ESG (Environmental, Social, Governance) goals. | Green computing, AI for sustainability |
Smaller Carbon Footprint | Uses cleaner data centers and eco-friendly chips to minimize environmental impact. | Carbon-neutral AI, clean energy AI |
Edge Device Compatibility | Lightweight models can run on smartphones, IoT devices, and low-power hardware without GPUs. | AI at the edge, low-resource AI deployment |
Global Accessibility | Makes AI tools usable in developing regions with limited computing power or unreliable electricity. | Inclusive AI, democratized AI, accessible machine learning |
Ethical AI Development | Aligns with ethical AI frameworks by reducing negative environmental impacts and promoting fairness. | Responsible AI, ethical tech, sustainable ethics |
Future-Ready Scalability | Enables long-term growth without exhausting resources or increasing emissions. | Scalable AI, sustainable innovation |
Encourages Innovation | Drives new research in neuromorphic computing, federated learning, and low-footprint algorithms. | Green AI research, innovative ML architecture |
Improves Corporate Image | Companies adopting Green AI benefit from better branding, PR, and consumer trust. | Eco-conscious brand, green enterprise, sustainability leadership |
Real-World Use Cases of Green AI
Green AI is already delivering sustainable results across diverse industries. These real-world applications show how environmentally conscious artificial intelligence is reshaping how we live, work, and innovate.
1. Agriculture: Precision & Sustainability
Use Case: AI-powered precision farming helps optimize water usage, fertilizer application, and pest control.
- How It Works: Green AI analyzes satellite images, drone footage, and soil sensor data to detect crop health and forecast yields.
- Impact: Reduces waste, improves food production efficiency, and promotes climate-resilient agriculture.
2. Transportation: Smarter Mobility
- Use Case: Optimizing traffic flows and electric vehicle (EV) logistics with AI.
- How It Works: Machine learning models improve route planning, charging station placement, and fleet energy efficiency.
- Impact: Cuts fuel consumption, lowers carbon emissions, and makes urban mobility more efficient.
3. Smart Buildings: Automated Energy Efficiency
- Use Case: AI manages lighting, heating, and cooling in homes and commercial spaces based on real-time usage.
- How It Works: Green AI uses IoT sensors and machine learning to reduce energy consumption during low occupancy times.
- Impact: Saves electricity, reduces emissions, and enables green architecture.
4. Data Centers: Greener Digital Infrastructure
- Use Case: AI controls cooling systems, server load balancing, and resource allocation.
- How It Works: Algorithms optimize energy use based on performance needs and environmental conditions.
- Impact: Reduces electricity costs and carbon footprints of AI training and storage facilities.
5. Scientific Research: Low-Energy Computing
- Use Case: Green AI models help simulate climate predictions, bioengineering, and materials discovery more efficiently.
- How It Works: Lightweight neural networks and low-resource machine learning reduce compute cycles for complex simulations.
- Impact: Supports environmental science with less environmental cost.
Green AI is more than a buzzword—it’s a necessary shift toward responsible innovation. These real-world applications prove that artificial intelligence can be powerful and planet-friendly at the same time.
Green AI Is the Future We Need
Green AI is about more than saving energy—it’s about building intelligent, ethical, and sustainable technology for the long term. Whether you’re a developer, business leader, or consumer, embracing Green AI means choosing innovation that aligns with the planet.
Ready to build a smarter, greener AI system? Contact experts in a sustainable AI development company today and join the movement toward a cleaner digital future.