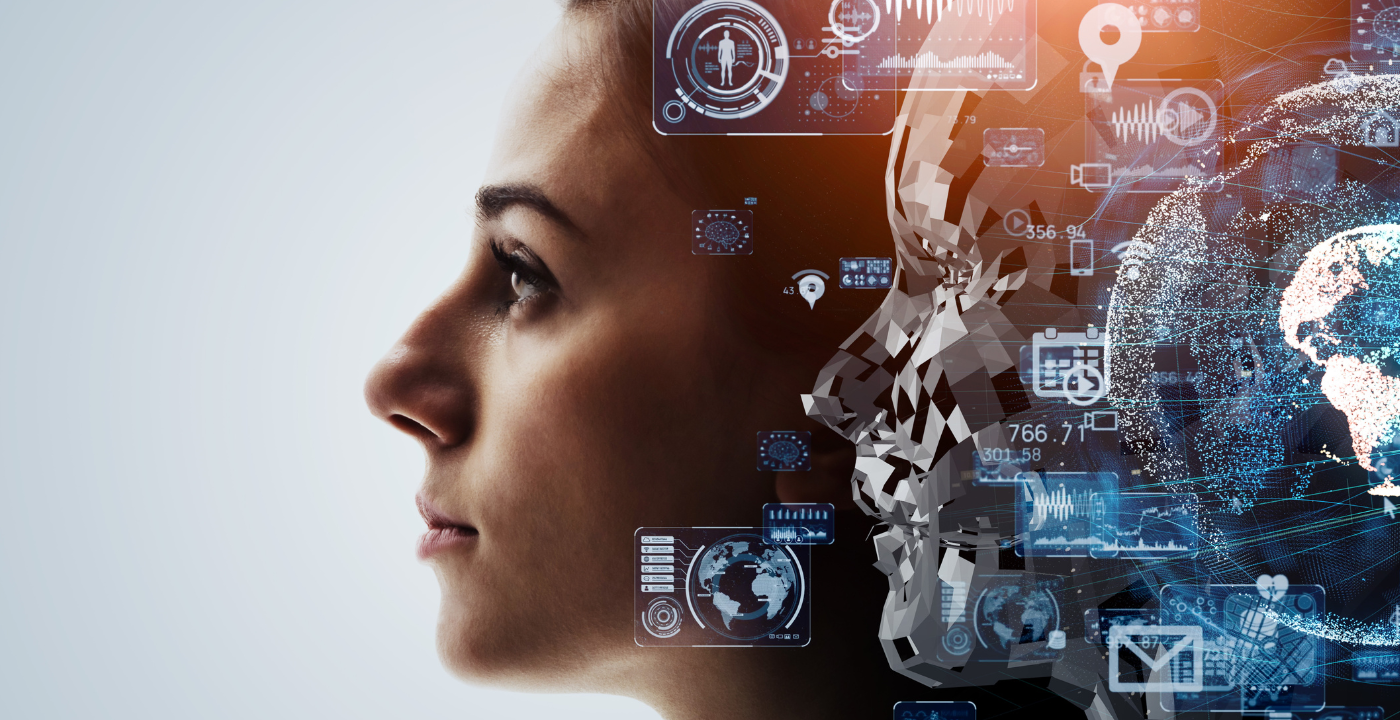
Generative AI is not the invention of a single individual but rather the result of collaborative efforts and advancements in the field of artificial intelligence (AI) and machine learning (ML) over several decades. It is important to note that generative AI, which involves the generation of content, data, or information, has evolved from various AI and ML techniques and models developed by numerous researchers and organizations.
Generative models like Generative Adversarial Networks (GANs) and recurrent neural networks (RNNs), which are fundamental to generative AI, have been developed and refined by a community of researchers, and their contributions span multiple years and institutions.
One of the notable advancements in generative AI is the development of deep learning techniques, which have greatly enhanced the capabilities of generative models. The concept of deep learning, involving neural networks with multiple layers, has significantly contributed to the success of generative AI models.
While there isn’t a single inventor of generative AI, it is a field that continues to advance through the collaborative efforts of researchers, engineers, and organizations worldwide. Prominent organizations like OpenAI, Google, and universities around the world have played significant roles in pushing the boundaries of generative AI and bringing it to the forefront of AI research and applications.
Who Invented Generative AI?
Generative AI, the field of artificial intelligence focused on creating new data, has become a revolutionary force. From creating realistic images to composing music, generative models are pushing the boundaries of what machines can achieve. But who gets the credit for inventing this transformative technology?
Generative AI: A Collaborative Effort
The truth is, generative AI isn’t the brainchild of a single inventor. It’s the culmination of decades of research and development by countless mathematicians, computer scientists, and artists. The field has seen significant contributions from various areas, each laying the groundwork for the next breakthrough.
Early Seeds: From Science Fiction to Theory
The concept of machines capable of creative generation can be traced back to science fiction. Early works like Mary Shelley’s Frankenstein explored the idea of artificial beings. However, the scientific exploration of generative AI began in the mid-20th century.
- Alan Turing (1950s): A pioneer in computer science, Alan Turing’s 1950 paper “Computing Machinery and Intelligence” introduced the Turing Test, a thought experiment to determine a machine’s ability to exhibit intelligent behavior. This concept laid the foundation for exploring the possibility of machines capable of creative thought.
Statistical Techniques and Language Modeling
The early days of generative AI invention development saw the use of statistical techniques to model and generate data.
- Andrey Markov (Early 1900s): The work of Russian mathematician Andrey Markov on Markov chains, which analyze sequences of events, became a foundational concept for language modeling. By analyzing patterns in text, Markov chains could be used to generate new, but statistically probable, sequences of words.
The Dawn of Artistic AI (1960s-1970s)
As computer technology advanced, artists began exploring the potential of AI for creative expression.
- Harold Cohen (1970s): Harold Cohen, a British artist, developed AARON, a computer program that could generate abstract art. AARON used a set of rules and procedures to create unique and ever-evolving drawings, showcasing the potential for AI-driven artistic exploration.
The Rise of Machine Learning (1980s-2000s)
The development of machine learning algorithms, particularly artificial neural networks, significantly impacted generative AI.
- Recurrent Neural Networks (RNNs) (1980s): RNNs, a type of neural network capable of processing sequential data, became instrumental in natural language processing (NLP) and text generation. By analyzing sequences of words, RNNs could learn the structure of language and generate grammatically correct sentences.
- Long Short-Term Memory (LSTM) Networks (1997): A specific type of RNN, LSTMs addressed the vanishing gradient problem, allowing RNNs to learn long-term dependencies in data. This advancement significantly improved the quality of generated text.
Generative Adversarial Networks (GANs) and the Deep Learning Revolution (2014-Present)
The introduction of Generative Adversarial Networks (GANs) by Ian Goodfellow in 2014 marked a significant leap forward in generative AI.
- Generative Adversarial Networks (GANs) (2014): GANs involve two neural networks – a generator and a discriminator – locked in an adversarial training process. The generator creates new data, while the discriminator tries to distinguish real data from the generated data. This competition pushes both networks to improve, leading to increasingly realistic and sophisticated generated outputs.
The success of GANs, coupled with the advancements in deep learning, has fueled the rapid development of generative AI in recent years. We’ve seen breakthroughs in:
- Image Generation: Creating realistic and photorealistic images of objects, faces, and scenes.
- Text Generation: Generating different creative text formats, like poems, code, scripts, and musical pieces.
- 3D Modeling: Creating new 3D models of objects and environments.
The Future of Generative AI: Collaboration and Continued Innovation
Generative AI is still in its early stages, but its potential is vast. As research continues, we can expect even more sophisticated and impactful applications. The future of generative AI likely lies in continued collaboration between researchers, engineers, and artists.
Here are some exciting possibilities for the future:
- Personalized experiences: Generative AI can personalize content, products, and services based on individual preferences.
- Scientific discovery: AI can assist in drug discovery, material science research, and other scientific fields by generating new possibilities and hypotheses.
- Enhanced creativity: Generative AI can be a powerful tool for artists, musicians, and writers to explore new creative avenues.
To know more about how a Generative AI can help your business, talk to Vegavid Generative AI Development Company.