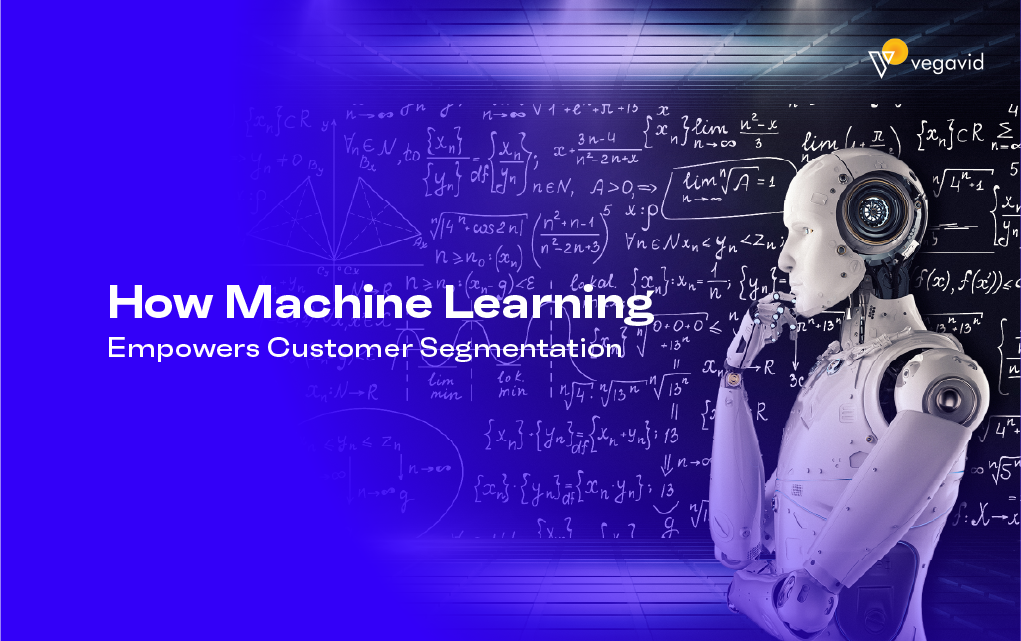
Today in our data-driven business environment, knowing who your customers are is important. Although traditional methods of segmentation can be useful, they often fail to bring out the intricate relationships that exist within large customer datasets. This is where Machine Learning (ML) comes into play, providing a very powerful alternative for seeing hidden patterns and developing highly targeted customer segments.
This blog post looks at Machine Learning for customer segmentation as well as its benefits, tools, and practical aspects. After reading this article you will be able to understand how ML can help uncover deeper insights about your customers and enable you to create personalized marketing approaches which will resonate with them.
Why Use Machine Learning in Customer Segmentation?
One of the most important processes in marketing—customer segmentation—is the division of customers into groups based on their shared attributes. This allows businesses to customize their messages, products, or services according to specific consumer needs resulting in increased interaction levels and conversions.
Traditional approaches often use demographic factors such as age, income, and location. Nonetheless, these variables are not sufficient by themselves. However, Machine Learning overcomes these limitations by:
- Unearthing Hidden Trends: The ML algorithms can scan through substantial amounts of customer data comprising purchase history, web behavior, and social media engagements among other things. This way, it brings to light hidden trends and associations that may go unnoticed by traditional methods.
- Dynamic Segmentation: Consumer activities change with time. Thanks to machine learning models, you can keep your segmentation up-to-date.
- Predictive Power: ML predicts what people will do next so that you can focus on the ones who are likely to become customers or leave before they even get started.
Revealing Enigma: Important Machine Learning Techniques for Segmentation
In the domain of ML, there is a wide range of possibilities in terms of tools used for customer segmentation. Some examples include:
- K-means Clustering: This unsupervised learning technique reveals groups (clusters) in your data based on pre-defined attributes. Consider dividing customers according to their buying patterns and demographics. K-means repeatedly assigns points to their closest cluster which shows different customer segments.
- Hierarchical Clustering: This unsupervised type forms a tree-like hierarchy of clusters. Such analysis allows one to approach consumer segments at different levels of granularity offering a flexible view of the target audience composition.
- Latent Dirichlet Allocation (LDA): It is aimed at text categorization of things such as words or documents especially when dealing with customer information.
Choosing the Right Technique:
The optimal ML technique depends on your specific data and segmentation goals. Here are some factors to consider:
- Data Type: Are you dealing with numerical data (purchase history) or textual data (reviews)?
- Segmentation Objectives: Do you want to identify high-value customers, predict churn risk, or personalized product recommendations?
- Data Availability: Choose a technique suited for the volume and variety of customer data you have access to.
Beyond the Algorithm: Considerations for Successful ML Segmentation
While ML unlocks powerful capabilities, successful customer segmentation requires more than just algorithms. Here’s what to keep in mind:
- Data Quality: The foundation of good segmentation lies in high-quality, accurate customer data. Ensure your data is cleansed and preprocessed to avoid introducing bias into your ML models.
- Feature Engineering: Selecting the right features, and data points that represent customer characteristics, is crucial. Choose features that are relevant to your segmentation goals and provide a holistic view of the customer.
- Model Evaluation: After building your ML model, assess its performance. Use metrics like silhouette score (clustering) or accuracy (classification) to ensure the model effectively segments your customers.
Real-World Applications: How Businesses Leverage ML for Segmentation
Imagine a streaming service using ML to segment customers based on viewing habits. They could identify high-engagement viewers, recommend personalized content, and offer targeted promotions, resulting in increased customer satisfaction and retention.
Retailers can leverage ML to segment customers based on purchase history and predict churn risk. By proactively engaging at-risk customers with personalized offers, they can minimize churn and drive customer loyalty.
The possibilities are vast. Travel companies can personalize recommendations based on past travel preferences, while banks can create targeted financial products for specific customer segments.
Conclusion: Embrace the Power of Machine Learning for Smarter Segmentation
In today’s competitive landscape, understanding your customers is more critical than ever. Machine Learning empowers you to go beyond demographics and delve into the rich tapestry of customer data. By leveraging ML for customer segmentation, you can unlock a deeper understanding of your customer base, personalize your marketing strategies, and build stronger customer relationships.
As with any technology, ethical considerations are important. Ensure your ML models are unbiased and respect customer privacy regulations.